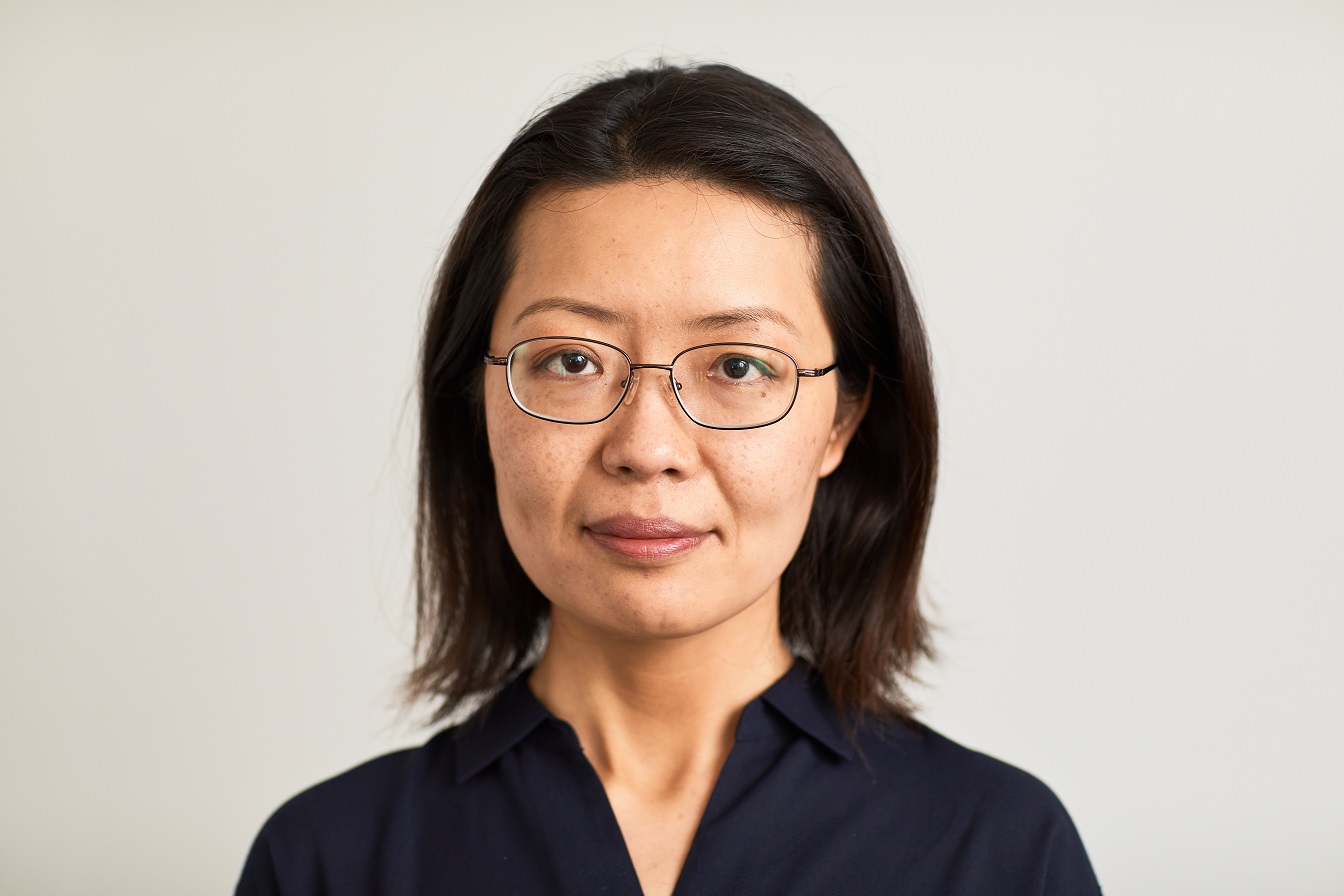
Keywords
Fields of Research (FoR)
Computer vision, Deep learning, Pattern recognition, Machine learning, Applications in health, Image processing, Social robotics, Graph, social and multimedia dataBiography
Dr Yang Song is an ARC Future Fellow and Scientia Associate Professor in the School of Computer Science and Engineering. She is also currently the Associate Head of School (Research). She graduated with a BEng in Computer Engineering from Nanyang Technological University, Singapore, and obtained a PhD degree in Computer Science from the University of Sydney in 2013.
Her research area is in Computer Vision, Biomedical Image Analysis, Machine...view more
Dr Yang Song is an ARC Future Fellow and Scientia Associate Professor in the School of Computer Science and Engineering. She is also currently the Associate Head of School (Research). She graduated with a BEng in Computer Engineering from Nanyang Technological University, Singapore, and obtained a PhD degree in Computer Science from the University of Sydney in 2013.
Her research area is in Computer Vision, Biomedical Image Analysis, Machine Learning, Deep Learning, and AI. She has over 220 peer-reviewed publications including papers in TMI, MedIA, TIP, TMM, NeuroImage, Bioinformatics, CVPR, ICCV, AAAI, IJCAI, ICLR and MICCAI. Her current research mainly focuses on the development of machine learning and deep learning algorithms for computer vision problems such as:
- Segmentation in radiological images
- Cancer analysis in histopathology images
- Cell segmentation and tracking
- Point clouds analysis
- 3D image reconstruction
- Object detection and recognition
- Action recognition and video analysis
- Vision-based autonomous driving
- Image enhancement and translation
Personal website - http://www.cse.unsw.edu.au/~ysong/
My Awards
Selected awards:
- 2024 ARC Industrial Transformation Research Hub for Human-Robot Teaming (2024 - 2029)
- 2023 Award for Inclusion Research, Google
- 2023 ARC Linkage Project (2023 - 2026)
- 2023 Women in AI 2023 Asia-Pacific Award in AI in Innovation
- 2022 NHMRC Ideas Grant (2023 - 2027)
- 2021 Faculty of Engineering Research Excellence Award, UNSW
- 2021 Impact Scholar, Google
- 2020 Scientia Fellowship, UNSW (2020 - 2028)
- 2019 ARC Future Fellowship (2020 - 2024)
- 2017 Dean’s Research Award, University of Sydney
- 2015 ARC Discovery Early Career Researcher Award (DECRA) (2015 - 2018)
- 2013 Google Publication Prize
My Research Supervision
Areas of supervision
Key areas: biomedical image analysis, general computer vision, deep learning, machine learning, interdisciplinary computer vision applications, multimedia data analysis
Actively recruiting PhD students. Interested candidates are strongly encouraged to apply.
See my personal website for more information - http://www.cse.unsw.edu.au/~ysong
My Teaching
- COMP9517 - Computer Vision
- COMP9417 - Machine Learning and Data Mining
- COMP9491 - Applied Artificial Intelligence
- Vertically Integrated Project - AI 4 Everyone
- Supervision of Honours thesis studies and Master's research project studies
Publications
ORCID as entered in ROS
