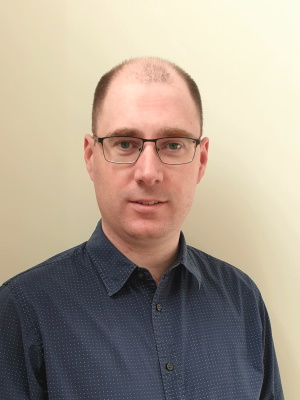
My Expertise
Digital viticulture, horticultural sensing, image processing, autonomous systems, 3D mapping, unmanned ground vehicles, robotics
Fields of Research (FoR)
Horticultural crop growth and development, Oenology and viticulture, Automation engineering, Autonomous vehicle systems, Field robotics, Autonomous agents and multiagent systems, Intelligent robotics, Computer vision, Image processingSEO tags
Biography
Mark is an Adjunct Senior Lecturer in the Mechatronics discipline within the School of Mechanical and Manufacturing Engineering. Please see his research group's page at www.robotics.unsw.edu.au/srv for more details of the work his team has done. His research interests were focussed around digital agriculture and digital viticulture and extend to the following topics, however he is no longer working as a full time academic:
- Yield estimation...view more
Mark is an Adjunct Senior Lecturer in the Mechatronics discipline within the School of Mechanical and Manufacturing Engineering. Please see his research group's page at www.robotics.unsw.edu.au/srv for more details of the work his team has done. His research interests were focussed around digital agriculture and digital viticulture and extend to the following topics, however he is no longer working as a full time academic:
- Yield estimation and data mining in precision viticulture
- Digital agriculture including apple flower density estimation
- Digital viticulture including smartphone app development
- Agricultural robotics
- 3D point cloud processing
My Grants
Industry Engagement
- Consultant to NXTGen Industries for "Review of mechanical, electrical and system design for a robot for bridge maintenance" (2021), value $5,000
- Chief Investigator for contract research with Plant and Food Research New Zealand, "Embedded Metadata" (2021), value $9,000.
- Chief Investigator for contract research with Green Atlas, "Image Processing in Orchards" (2020), value $5,000.
- Chief Investigator for contract research with Plant and Food Research New Zealand, "Semi-automated 3-D and multispectral bunch sorting technologies" (2020), value $50,000.
- Partner Investigator for contract research with Plant and Food Research New Zealand, "Hyperspectral imaging of grapevines for spray deposition analysis" (2018 – 2021), value $30,000.
- Chief Investigator for contract research with Lincoln Agritech, "Grape Yield Analyser Project" (2019), value $5,000.
- Partner Investigator for contract research with Plant and Food Research New Zealand, "Geospatial resolution in vineyards: Project Demonstrator 8008" (2018), value NZD$10,000.
- Chief Investigator for contract research with Pernod Ricard, "Data mining for viticultural yield estimation" (2018), value $24,000.
- Chief Investigator for contract research with Dematic, "Computer Vision for Rack Inspection" (2017), value $65,714.
Research Grants
- Partner Investigator for South Australian Wine Industry Development Scheme, "Using a camera system to track Eutypa in vineyards" (2021-2022), value $173,000.
- Partner Investigator for HIA funded Matched Levy Funded Investment Pool and Strategic Coinvestment Pool, "Developing Agri-tech solutions for the Australian apple industry" (2018-2021), value $4,004,000.
- Project Supervisor for contract research with Khalifa University, "MBZIRC2020 research grant" (2018 – 2020), value USD$50,000.
- Project Supervisor for AGWA funded Ph.D scholarship project “Autonomous In-Vivo Determination of Maturity Parameters” (2016 – 2018), value $30,000.
- Chief Investigator for Innovation Connections grant with Makinex "Development of an autonomous concrete cutting robot" (2017), value $99,700.
- Chief Investigator for AGWA (Australian Grape and Wine Authority – also known as Wine Australia) (Cat. 1) funded project “Improved Yield Estimation for the Australian Wine Industry” (2014-2017), value $819,000.
- Partner Investigator for AGWA (Cat. 1) funded project “Smart-Phone Based Image Analysis to Assess Vine Water Stress” (2016 – 2017), value $332,400.
- Partner Investigator for UNSW Strategic Educational Development Grants #2, “Interactive learning modules for Engineering fundamentals” (2014 - 2015), value $300,000.
- Chief Investigator for CRC-SI (Cooperative Research Centre for Spatial Information) funded project “Identification of indoor localisation solutions for tracking patients in hospital environments to minimise cross-infection” (2016), value $24,500.
- Academic Supervisor for NSW Government Research Attraction and Acceleration Program grant to MBZIRC team, “Science and Engineering Student Competition Sponsorship Program” (2016), value $15,000.
- Chief Investigator for AGWA funded travel bursary “Study tour to support automated yield estimation project” (2015), value $1,500.
- Academic Supervisor for NSW Government Research Attraction and Acceleration Program grant to IGVC team, “Science and Engineering Student Competition Sponsorship Program” (2015), value $8,000.
- Partner Investigator for DAAD research grant to collaborate with the University of Oldenburg “Development of Methodologies to Actively Compensate for Erratic Motions in Micro/Nano Manipulators” (2013-2014), value $20,000.
My Qualifications
- 2015 Graduate Certificate in University Learning and Teaching at UNSW Australia.
- 2008 - 2012 PhD, Mechatronic Engineering at the University of New South Wales - link to dissertation titled "Efficient path planning in deformable dense maps."
- 2003 - 2007 BE (Mechanical) / BSc (Computer Science) at the University of New South Wales
My Awards
- Academic Supervisor for UNSW's Competitive Robotics Group, awarded equal second place in Challenge 3 of the 2020 Mohamed bin Zayed International Robotics Challenge.
- Supervisor and co-author of Best Student Poster at AWITC2019 and AWITC2016.
- Faculty of Engineering Staff Excellence Award for Research Excellence (2016)
- Academic Supervisor for New South Solutions, UNSW's entry in the 2015 Telstra University Challenge. Top three teams in Australia for their sun exposure tracking solution. Video.
- Academic Supervisor for Saving Allen, UNSW's entry in the IGVC2015 event. Won best UGV performance, breaking all course records.
- Academic Supervisor for UNSW Mechatronics Team 2. Won the grand prize in the AGVC2014 event. Featured on ABC TV and online news.
- Academic Supervisor for UNSW AGVC Team 2. Won second place overall and and Best Autonomous Vehicle performance at the inaugural Autonomous Ground Vehicle Competition in December 2013
- Winner, Student Reporter Contest, ICRA2011, with two articles published in the IEEE Robotics and Automation Magazine (2001)
- William McIlrath Postgraduate Travel Scholarship (2010)
- Dean’s Award for Excellence in Postgraduate Research, Best Overall Student (2010)
- Best Paper and Best Presentation award, 4th US-European Workshop on Micro Aerial Vehicles (IMAV09), Pensacola, Florida (2009)
- UNSW Research Excellence Award for postgraduate study (2008 - 2011)
- Senior Bachelor of Engineering Award, Engineers Australia, Sydney Division (2008)
- University Medal for Mechanical Engineering (2007)
My Research Activities
- Co-chair of Agriculture and Environment group, National Robotics Network (2020 - 2021)
- Treasurer of Australasian Robotics and Automation Association (2017 - 2019)
- Co-chair of 2019 AGRICONTROL conference, held in Sydney from 4-6 December 2019
- Local chair for the 2013 Australasian Conference on Robotics and Automation, held at UNSW in December 2013
- Secretary of Australasian Robotics and Automation Association (2013 - 2016)
- Organiser of the workshop on Recent Advances in Agricultural Robotics, co-located with the 13th International Conference on Intelligent Autonomous Systems (2013)
- IEEE RAS Agricultural Robotics and Automation technical committee member (2014 - 2022)
- IEEE Robotics and Automation Society member (2011 - 2022)
- IEEE Member (2008 - 2022)
- IFAC 8.1 Technical Committee on Control in Agriculture member (2019 - 2021)
My Research Supervision
Areas of supervision
I am not seeking further students.
Currently supervising
Current Students and Topics
Ph.D
- Mengying Hu: Canopy detection for apple spraying robot
Completed Students and Topics
- Annie Xu Wang: Flower detection for apple spraying robot
- Bolai Xin: 3D grape bunch reconstruction
- Julie Tang: Vine bunch maturity estimation using multispectral cameras
- Masami Hirayama: Autonomous operation of bulldozers for ground levelling (co-supervised with Jay Katupitiya and Jose Guivant as primary supervisors)
- Scarlett Liu: Yield Estimation in Viticulture by Digital Image Processing
M.Res
- Cameron Stewart: Improving estimation of distributions (co-supervised with Alan Blair as primary supervisor)
- Joshua Purnell: Design of an actuated towed underwater glider (with Ocius)
- Dylan Sanusi-Goh: Mapping road condition from bus sensor data
- Raghav Hariharan: Improving row detection in horticultural crops
- Olivia Yem: Apple fruitlet recognition
- Rowan Ramamurthy: Autonomous 3D reconstruction of towers (with Emesent)
- Gilbert Rayner: Green on green weed detection
- Michael Kedar: Grape bunch reconstruction from MicroCT scans
- Timothy Ryan: Post and tree detection from LiDAR
- Henry Phung: GPU speed-ups for bulldozer planning algorithm
- Sophia Lin: User interface for an app for grapevine dieback detection
- William Chen: Improving the accuracy of plane SLAM
- Patrick Moulds: Robot grasp training by gesture recognition
- Dominic Daners: Design and construction of an swerve drive platform
- Liangde Li (with Claude Sammut): Robocup - GPU processing
- Oliver Richards (with Claude Sammut): Robocup - semantic tracking of objects
- Leandro Hirai: GANs for augmenting training data for image segmentation
- Rebecca Schacht: Task allocation for multi-robot exploration (co-supervised with CSIRO)
- Elliott Smith: RRTs for automated bulldozer path planning
- Anthony Feizi-Sobbi: UAV real-time image processing
- Edward Thomson: UAV real-time image processing
- Kenji Brameld (with Claude Sammut): Robocup - fall recovery
- Adam Tizzone: Localising UAVs from onboard sensors
- Marcus Swift: Bulldozer path planning in constrained situations
- Aaron Spyrakis: App for delivery drivers
- Sarah Howells: Image processing for vine row detection
- James Sloan: Speeding up neural networks on quantum computers
- Yiwei Han: 3D Reconstruction of Grape Bunch Rachis
- Gabriel Low: Grape Detection
- Luke Millstead: A Fast Method for the Calculation of Stomatal Conductance
- Benjamen Lee: Implementation of a Storage Optimised and Flexible Blockchain
- Carson Crosse: 3D Model of Grape Rachis Structure from Video
- Albert Chau: Vertical farming: a review
- Linda Wong: Investigating the Resource-Constrained Project Scheduling Problem via Quantum Computing
- Jabez Wilson: Visual Inertial Odometry on an Unmanned Aerial Vehicle
- Scott Bennett: Using Machine Learning to Investigate Trends in the Dispensing of Dementia Medication
- Kevin Muliady: Using Convolutional Neural Networks to detect the shoreline in beach images
- Sean Thompson: Narrative Generation
- Rafael Lawrence: Impact of visual feedback on chord typing
- Tom Wyatt: Rural Mobile Networks
- Nicola Gibson: Real-time Filtering of LiDAR Point Clouds Corrupted by Rain
- James Murdoch: Reinforcement learning for optimal control of a Reverse Osmosis plant
- Harsh Patel: Automated Image Stitching and Classification for Vine Water Stress (VWS) Project
- Phillip van Kerk Oerle: Towards Better Grape Yield Estimation using Convolutional Neural Networks
- Joseph Salim: Development of a Novel Sensor to Detect Incipient Slip and Estimate Coefficient of Friction
- Alex Gan: Location Prediction using a region-based Hidden Markov Model
- Ian Stuart Bartlett: Training of Conditional Restricted Boltzmann Machines on a Quantum Computer
- William Wu: Database for Collating Indoor Localisation Records
- Faris Azhari: Particle Filter Methods for Indoor Localisation using Mobile Phones and Bluetooth Beacons
- Drew Wiseham: Yield Estimation in Viticulture
- Zhen (Steven) Liu: Berry Diameter Tracking
- Gaurav Awadhwal: Improved Camera and GPS Data Capture for Yield Estimation
- Christopher Squire: Improving Point Cloud Rendering with Skip-octrees
- Amogh Sarda: Natural Language Processing for Predicting Self-harm in Online Forums
- Laura Hodges: Agent-based Task Allocation and Exploration for UAVs
- Lalitha Katupitiya: Centralised UAV Coordination
- Fredrik Westling: Mobile Phone Control of a Quadcopter
- Cameron Stewart: Deep Neural Networks on the D-Wave 2X Quantum Computer
- Kevin Lerdwichagul: AI for Starcraft
- Shirleen Sharma: Believability of Conversational Agents in Role Playing Games
- Cadmus To: Pose Estimation for Grasping Unknown Objects
- Sang-woo Park: UGV Manipulator Design
- Daniel Ly: 3D Object Pose Tracking using a Kinect Sensor
- Karthik Jayaraman: Indoor Localisation in a Hospital Environment using Bluetooth Low Energy Tags
- Terence Chong: Generation of Virtual Formula SAE Racing Environments using Road Scanning Systems
- Thomas Wijaya: Geometric Consistency and Optimisation for Map Merging
- Phillip Moser: Automated Sorting System for a Grape Harvester
- Will Russell: Inducing Cow Flow in Grazing AMS Dairy Farms Using Audio Stimuli
- Nathan Adler: Development and Assessment of a Low Cost CORS-linked Single Frequency GPS for RTK Applications
- Rory San Miguel: Design of a High Precision UAV GPS
- Andrew McGill: Development of a Smartphone Application to assist in Viticulture Data Collection
- Sam Cassisi: Hospital Patient Tracking for Infectious Disease Control
- Zhu (Arthur) Lie: Mobile Phone Control of a Mobile Robot
- Sudeep Singh: Automated Image capture for Grape Vine Inspection
- Samuel Binns: Robot Manipulator Grasp Planning
- Lindsay Folkard: Visual Based Egomotion Detection
- Stephanie Morris: Inflorescence Counting using Image Processing
- Tanawat Sarnvanichpitak: Defect Detection in a Coat Hanger Manufacturing Line
- Julie Tang: Automated Screw Detection and Removal for Robotic Disassembly
- Ian Craig: Personal Location Prediction from Automatic Region Clustering using Mobile Devices
- Russell Harding: Mobile Phone Control of a Mobile Robot
- Letty Liu: Inflorescence Flower Counting by Image Processing
- Dan Padilha: Implementation of NP-Hard problems on the D-wave 2 quantum computer
- Samuel Marden: Automated Yield Forecasting in a Vineyard using an Unmanned Ground Vehicle and Computer Vision
- Aditya Pradiptadarma: Design and Evaluation of a Flexible Vineyard Management System
- Donger (Donald) Luo: Simulation Modelling of a Quadbike to Help Improve Stability
- Andrew Bastoli: Dynamic Memory Management of a Point Cloud Viewer
- Chloe Boyton: Automatic Generation of Aircraft Loading Schedules
- Chi Quan (Calvin) Tan: Plane Extraction and Visualisation in 3D Poinbt Clouds
- Kent Tran: Real Time Visualisation of Large Point Cloud Datasets
- Maximilien Stevens: 3D Map Merging of Campus Scale Datasets
- Sheng Jie Teo: Robot Controlled Pan and Tilt DSLR Camera over the Internet
- Tom Lindeback: Planning with Multiple Constraints
- Brett Seton: An Investigation into Path Planning Techniques
- Dylan Campbell: The Kidnapped Robot Problem: Detection and Recovery
- Paul Ashley: Development of Smartphone Applications in Viticulture
- John Goward: Estimating and Predicting Carbon Sequestered in a Vineyard with Soil Surveys, Spatial Data & GIS Management
- Cameron Joannidis (co-supervised with Steve Cossell): Implementation of an Audio Interface for the UNSW Unmanned Ground Vehicle
- Derek Taprell: Development of a Module Base Station System for a Micro Aerial Vehicle & The Investigation of a Laser Stripe Distance Sensor for a Micro Aerial Vehicle
- Finn Russell: Improved smartphone apps for vine trunk disease detection
- Yingtao Zhang: Apple flower counting
- Aaron (Shanda) Su: An online system for vine flower counting
- Luke Millstead: Microscope image processing
- Faris Azhari: Indoor Localisation using Mobile Phones and Bluetooth Beacons
- Yiwei Han: Mobile Computer Vision for Yield Estimation in Viticulture
- Zhihao Zhang: Georeferencing Vineyard Imagery Without GPS
- Xin Dong: Google 'Street View' for the Vineyard
- Julie Tang: Long Term Autonomy for Image Collection in a Vineyard using a Mobile Robot
- Letty Liu: Inflorescence Flower Counting by Image Processing
My Teaching
Lectures / Courses previously taught
- MTRN4230 Robotics (course convenor and lecturer)
- MTRN2500 (course convenor and lecturer)
- MMAN3000 Professional Engineering and Communication (lecturer)
- Previously: MMAN1300 (lecturer), MMAN2300 (lecturer), MTRN2500 (tutor), MTRN3500 (tutor), MTRN3020 (tutor), GSOE9400 (tutor), MMAN3200 (tutor), MTRN3100 (tutor), MTRN9222 (tutor), SENG3111 (tutor)
Publications
ORCID as entered in ROS

Videos

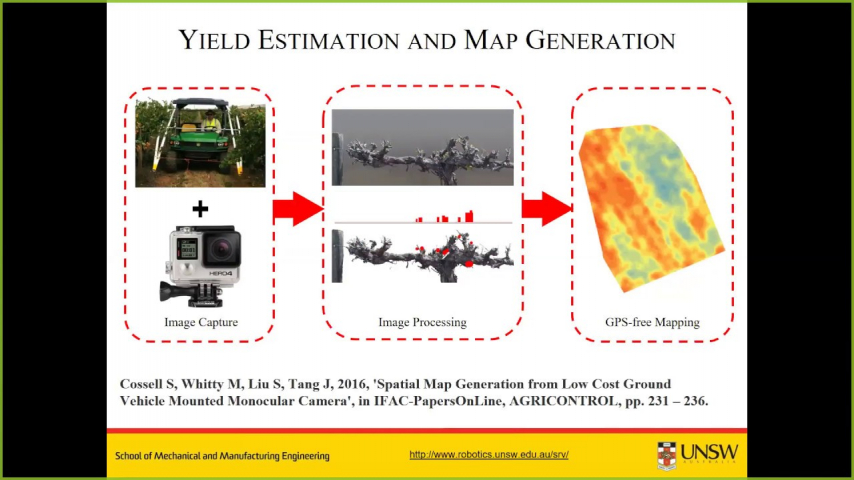
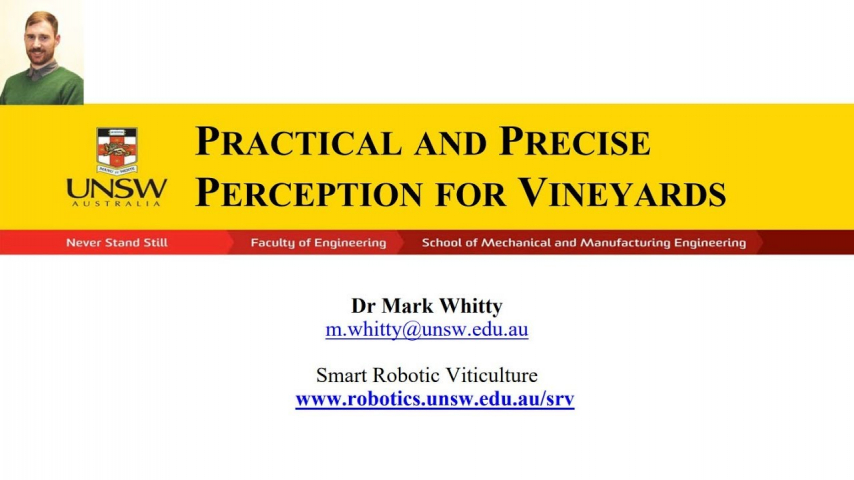
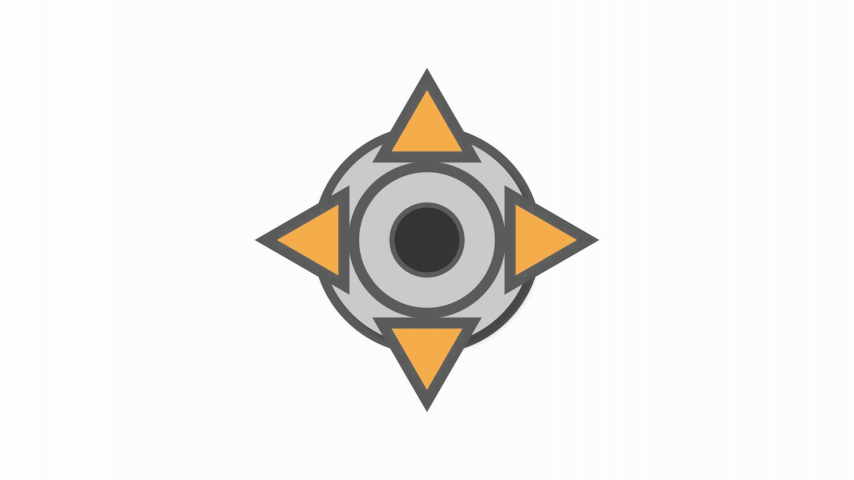
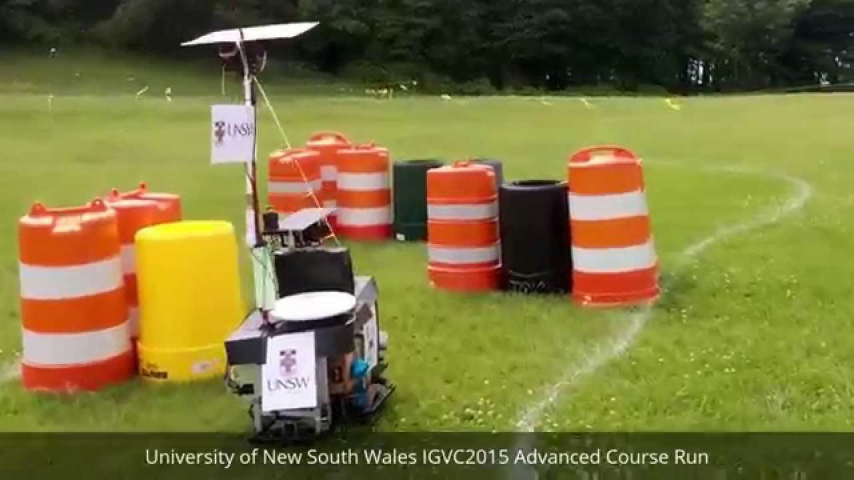
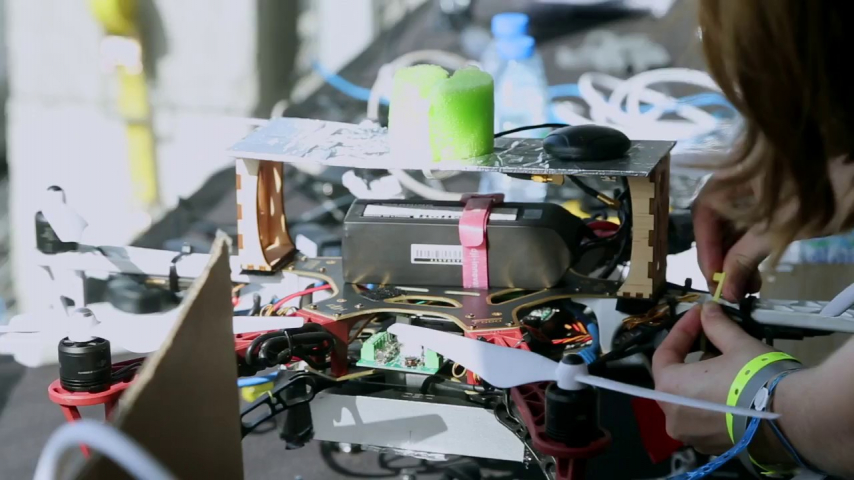
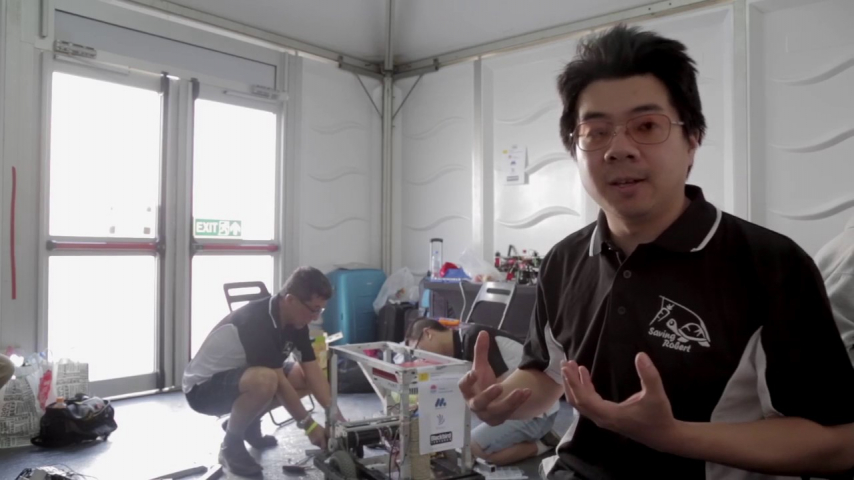
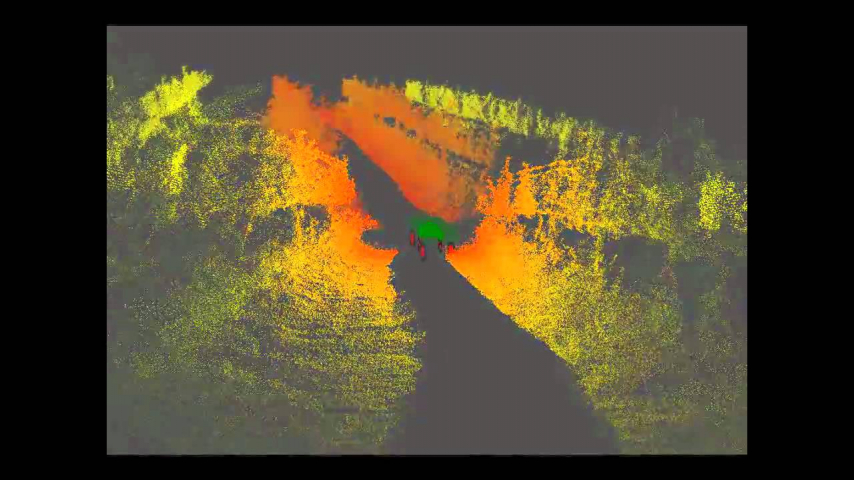
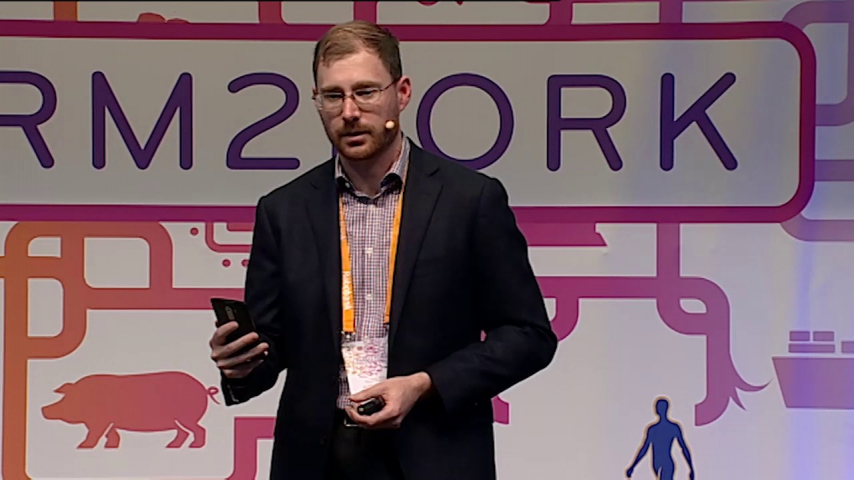